Before we get into the deets, here are the links if you want to check out what I built:
- ArtificialStupidity.org – the homepage
- The Glorious TED Talk Page
- The TED Talk Clip That Changed Nothing
- The Merch Store : (hat), (tshirt), (Phone Cover)
- The Twitter Feed
Yesterday I participated in a 90 vibe-marketing sprint competition. The challenge was to see what you could come up with in 90 minutes as a marketing campaign for an existing or a created product.
Before I began, I asked the organizers—twice—whether I should build something useful or just something cool. Someone told me, “Cool is useful, in marketing.” I don’t really agree with him. I still don’t. But I went for it anyway.
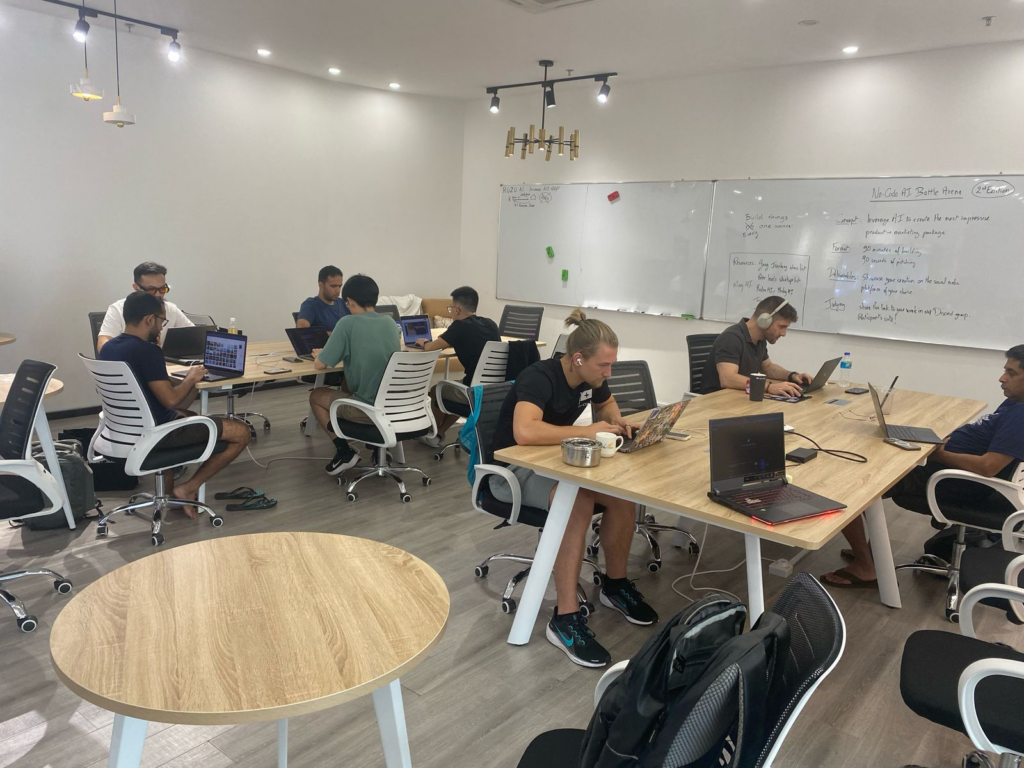
The gang
Earlier that week, I had trained a small, but cool image classifier to determine whether something was a bird… or not. It was my first time training an ML model so was very happy with it. I was proud.
The brand I decided to build was centered around the bird/not-bird classifier. A company that tries its best and comes up with not very helpful models but is very proud of the work they do. I don’t know, maybe it’s a reflection of how I feel here at NS. I am far behind most people here (at least technically), but I make things anyway and I think I’m improving (:
Yes, the universe is satire and takes itself too seriously, but I think I wanted to actually celebrate an entity just trying its best.
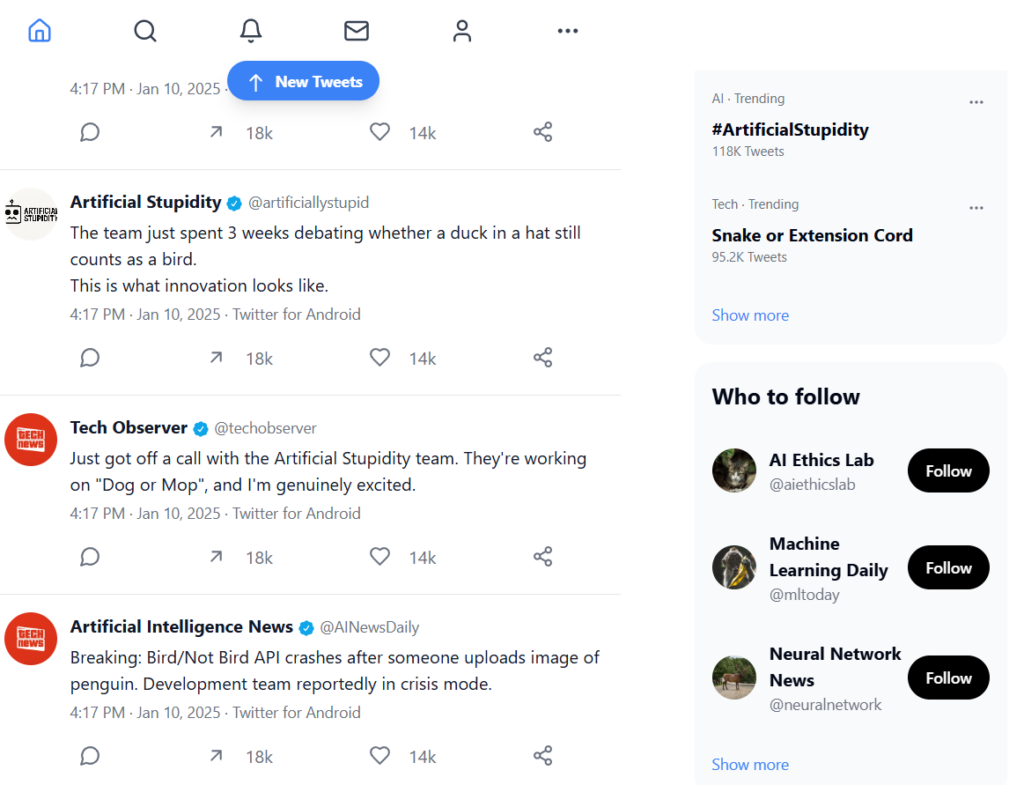
Courage, not accuracy
Anyway, enough moping about, over the 90 minutes had, I built a homepage and a brand identity, a fake TED talk and a TED page, and a merch store (complete with lore!).
But I think the thing I was most proud of was the Twitter feed.
For me, it was where the whole world of the project came to life. I built out a cast of fictional users, confused journalists, loyal customers, and rogue employees. People tweeting about how Bird/Not Bird misclassified their ex. News agencies reporting on our latest “breakthrough” with deeply concerning enthusiasm. Team members sharing proud screenshots of our tool misidentifying a cloud as “possibly bird.”
I couldn’t show most of it during the final presentation—I only got two minutes, and there’s no time in that to showcase a fake Slack meltdown about whether a hotdog counts as “bird-adjacent.” But it’s there, and it’s part of the experience.
Also: I recorded the entire process. All 90 minutes of it. From the moment I opened a browser to the last fake customer tweet. If you’re curious about how something like this gets made from nothing, I’m happy to upload it somewhere.
Karol, one of my favorite people at NS, called it a very high effort shitpost.
Fair.
(But fun).